Hannu Linturi, © Metodix O, originally published 12.6.2020, updated 6.2.2024
“A technique of structuring a communication process of a group in order to help to understand and deal with the future development of a complex problem.” (Linstone & Turoff 1975)
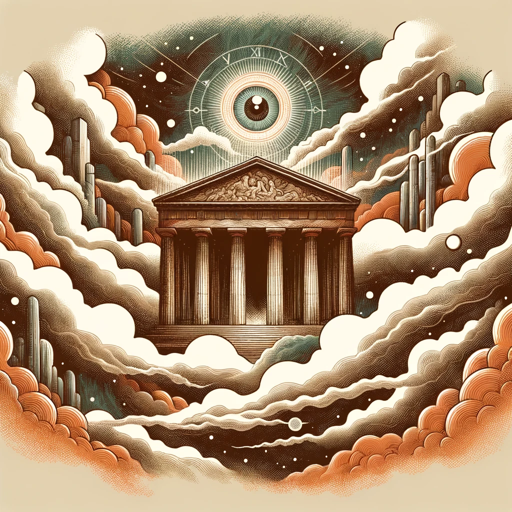
Delphi Oracle Bot https://chat.openai.com/g/g-O9I0o74MC-delphi-oracle
Three characteristics unify different versions of Delphi: anonymity, expertise, and iterativity. These characteristics have withstood the upheavals resulting from both technological development and expanding understanding of how knowledge is formed. Each characteristic has, in turn, also been questioned.
In the method blog series, nine articles will be published: (1) The Purposes of Delphi, (2) Steps of the Delphi Process, (3) Characteristics of Delphi Method, (4) The Delphi Panel, (5) The Delphi Manager, (6) Delphi Rounds (Iteration), (7) Delphi Method Connections, (8) Delphi Analysis (Future Mapping), and (9) The Future of Delphi.
The Delphi method can be characterized as a survey or interview-style research technique, where through a guided interaction process, there is an effort to gather and enhance the knowledge and understanding of a group of experts about the phenomenon under study. Reading between the lines of the definition, one can identify the most important and unifying characteristics of different Delphi method variations: the diverse expertise of the respondent group, the anonymity of commenting, and the recycling of knowledge formation among respondents. Together, these features lead to a research process that not only aims to collect existing knowledge but also strives to generate new knowledge.
These characteristics are not absolute, and each can be broken. Sometimes, stakeholder involvement may become more important than expertise. Anonymity can be abandoned if the parties represent established knowledge-producing entities whose positions and rationales are so permanent that the personal characteristics of the respondent are irrelevant. In recent years, one-round Delphis have become more common. The conditionality, even in basic features, makes Delphi an eclectic and flexible method, further emphasized by its use both qualitatively and quantitatively, and in some cases, both. The downside of this flexibility is that it exposes the method to criticism from different schools of thought.
Expertise and Stakeholder Involvement
Expertise is described as a learned and profound relationship with something, but at the same time, expertise is to a large extent a social construction. The Dictionary of Contemporary Finnish describes an expert as someone who has expertise, special, more thorough than usual (professional) knowledge from a certain field. The specifying descriptor from the dictionary fits well with the Delphi usage: ”specialist, expert, especially when called upon to give their opinion on a matter within their field.” This dictionary description is still static, but Wikipedia anticipates change.
The fracture is signaled by the fact that Wikipedia defines expertise but the Encyclopedia Britannica does not. Traditionally, Wikipedia starts from the premise that an expert is recognized by the mastery of reliable knowledge, technique, or skill. An expert differs from a specialist in that a specialist is capable of solving a problem, whereas an expert knows the solution. The opposite of an expert is a layman (layman) and of a specialist a generalist (generalist). Wikipedia also acknowledges that individuals are often experts in some areas and laymen in others.
Wikipedia does not yet take a stand on a more significant change in the concept of expertise, where expertise is no longer understood so much as a characteristic of the individual but of communities and networks. According to this view, knowing (knowledge formation) is fundamentally a social phenomenon, tied to cooperation, practices, and language. This development does not lead to the disappearance of expert knowledge but dismantles closed and hierarchical professions into multidisciplinary networks, where an important meta-property of expertise is the ability to connect with other expertises.
From the basis of collective knowledge conception, Delphi can be recommended as a tool for academic research as well as for learning communities and networks, for mapping future paths and choices. The communal approach is also a vaccination against the ossified form of expertise that Ivan Illich has described since the 1970s. Illich consistently criticizes an increasingly specialized society for separating most people from creative activity. A specialized and privileged social world order maintains a lack and dependency on the expert, who dominates the social discourse with their rhetoric. Illich demands peer and network activities in place of manipulative institutions, characterized by autonomy and interaction among people with their respective operational environments. This thinking has points of contact with Putnam’s theory of social capital.
Specialization and an advanced division of labor are recipes for success in the industrial society phase, emphasizing the importance of expertise. Modern expertise is inscribed with a hierarchy, which is concretized in social structures such as degrees and job titles. Social structures are reinforced by regulations and reward systems. In highly organized societies, the interests of different groups are looked after by unions and civil organizations. A large part of expertise is thus bound to interests, which can also be called stakeholder interests.
In a democratic society, each collective has its spokesperson, which enables a functioning negotiation system and stable societal development. The problem remains that not all collectives have an equally strong and organized voice, especially not those structures and actors that do not yet exist but could be in the future. The risk in a polarizing phase of societal development is that the balance among institutions representing different interests is disrupted, and the negotiation system drifts or is driven into crisis. As interaction decreases, the use of power becomes more monologic, and systemic understanding is simplified. The relationships between matters are increasingly seen as mechanical, unidirectional, and in accordance with the dominant discourse of power. These developments are entirely opposite to the ideas of the Delphi process.
Delphi is based, at best, on subjective knowledge. Even a successful Delphi does not tell us anything certain about the future. The research merely clarifies what experts think about the future. Humans cannot know in advance what scientific and technical inventions will be made in the future. Nor can we know how people will think and act tomorrow – what the operating environment and mindset in which the future human lives will be like. In this sense, there is no direct and unambiguous expertise available for the future. Therefore, it is wise to map out various possibilities and their probabilities and desirabilities.
Expertise always involves interests. There is, of course, a significant difference between the interest of a researcher and that of an actor who, for example, earns their living from the continuation of the current practice also in the future. In the latter case, the argumentation is predictable, but even then useful, because the argumentation arsenal of one option is certainly included in the examination. It is important to ensure that known interests are represented in the panel. More challenging is to find actors who give voice to things that have not happened, technologies that have not been realized, and social structures. Therefore, the panel often needs, in addition to experts and specialists, generalists (for the big picture of phenomenon development) and contrarians who challenge interest-driven expertise.
A Delphi panel invites a comprehensively diverse group of experts, which also representatively covers the interests, or stakeholder aspects, of the phenomenon under examination.
For the formation of communal knowledge, the most important meta-feature of the panel is a sufficiently open relationship to the future. Often, this requires projecting the future at least ten years ahead so that the immediate concentration of interests has time to dilute. The more stakeholder involvement there is in the panel, the further into the future the examination year should be moved. Conversely, an increase in the concentration of expertise has the opposite effect. Expertise may quickly thin out as technology and science progress. Technological development has other impacts, which can have their own paradoxical effects. One of them is that although network and digital technology has facilitated the implementation of Delphi, the number of personal expert interviews has increased. The social accessibility of experts may have potentially weakened. It remains to be seen what impact AI panelists formed by generative artificial intelligence will have?
Anonymity and Argumentation
Anonymity is a tool to liberate communication from the constraints produced by the social environment. The anonymity of experts avoids expression and listening constraints encountered in face-to-face groups. Through this, it aims to increase, diversify, and authenticate the argumentation of experts and stakeholders. In anonymous processes, a panelist’s status – high or low – does not influence other panelists’ opinions. Respondents do not need to fear losing face, even if they respond ”incorrectly” or comment ”loosely.” They also do not need to be wary of positions that their employer would not approve of publicly.
On the other hand, societal issues that are so well-established as phenomena that they can be discussed publicly are recognized. In these cases, there is a comprehensive variety of views on the phenomenon, each supported by an organized and knowledge-based entity. Petri Tapio (2002) questioned the significance of anonymity in his doctoral thesis on the future of transportation. In the study, anonymity was not significant because each participant was considered to represent a certain expert tribe united by a consensus on how to understand the phenomenon under study and its future.
Tapio’s model does not work as well when moving from transportation emissions to a social future whose potential institutions are still forming. How to study, for example, the future of learning where the learners are machines, through representation? It’s not impossible, as advocates of artificial intelligence are organized. Difficulties arise at the latest when a future party is not recognized at all.
A middle ground between anonymous and public appearances is the use of pseudonyms. The advantages of anonymity are retained, but the conversation can become personalized in a manner familiar from internet forums. The use of pseudonyms strengthens dialogues as panelists become personified and isolated comments evolve into lines of vision. The use of pseudonyms can create social structure, emotional energy, and group identity within the group. In some Delphi applications, such as xDelphi (www.xdelphi.ai), the emergence of dialogues is supported by a pseudonym practice, which does not reduce the benefits of anonymity.
In pedagogical Delphi exercises, roles have been used alongside pseudonyms. A role distances from uncertainty and enables immersion into play, which may become more ”real” than actual simulation. Positive experiences have been gained from method variations where initial rounds are conducted anonymously or with pseudonyms, but real names are used in the final rounds. This is especially the case when the group is already familiar with each other and accustomed to working together. A special case is when an expert panel is assembled for a common seminar before or after the actual rounds.
Anonymity is the default assumption of the Delphi process, but it can be bypassed for good reasons. In such cases, the panelist’s persona is not as important as the knowledge base they represent. In some Delphi variations, the use of a pseudonym or role adds value to the method.
One often-used motivational tool by managers is the knowledge of the exclusivity of the expert panel. Although the Delphi process is anonymous, participation in it is not. Knowledge about other participants interests the panelists. Notable participants attract others to join. Experts’ names and selection criteria are always listed in academic research reports, where the panel’s composition is a central methodological choice. Social attractors are important basic energy in the Delphi process. However, mere social attraction may not suffice to create a panel that learns to argue with each other. Ancient experts advised using all rhetorical qualities as catalysts for interaction: ethos, pathos, and logos. Logos, or reason, is of course the most important in Delphi, but it is ineffective without being empowered by deep feeling and noble aspirations.
Iteration, feedback, dialogue, learning
Delphi distinguishes itself from survey methods through its iterativity and feedback mechanism. Unlike in polls, opinions are not just collected for analysis, but the response data is recycled back to the experts. Through feedback, respondents are guided to justify their choices. Knowledge formation progresses in rounds so that the previous survey round forms the basis for the next one. The process resembles an ideal dialogue, as described by Martin Buber and Mikhail Bakhtin, among others. The dialogue aims to address disagreements without confrontation and to explore viewpoints one does not personally endorse.
The first round of questioning initiates the actual research process. It orients the research topic and helps respondents position themselves in relation to the phenomenon under study and to each other. Through comments and arguments, respondents clarify their positions and attempt to influence others’ perceptions. Panelists have the opportunity throughout the round to refine their responses. If this occurs, it is a positive signal of “listening” to other panelists and of dialogic potential. Between rounds, the manager analyzes the results and formulates new propositions from the presented arguments, which the panel then “votes” on in a new round.
In the internet age, Delphi allows for synchronous and real-time dialogue among experts. The first Delphi round may be structured into two phases, where an expert first responds to the survey and comments independently without seeing others’ responses. In the second phase, they are shown the distribution of responses and can comment on others’ comments. Panelists have the opportunity to argue against and support their own views as well as against others’ arguments, and as others have the same opportunity, the discussion branches and argumentation evolves already during the first round.
If real-time commenting and changing one’s own responses are allowed, the first Delphi round stretches to fulfill the traditional role of a second round. The difference from the classic second round is that the questionnaire remains unchanged. The second round is officially considered when the questions change, based on lifting the next round’s questions from the previous round’s arguments. Through this, the Delphi process can both deepen and broaden.
In web-mediated Delphi, it is possible to entirely abandon round segmentation. DARPA has developed the roundless Delphi tool, the Real-Time Delphi concept, for applications where immediate decision-making relevant information is delivered to a hypothetical decision-maker, simulating what Gordon & Pease (2006) describe. The roundless Delphi is also suitable for barometer-type Delphi applications used as qualitative forecasting tools. An example is the Finnish National Agency for Education’s Future of Learning 2030 barometer (see https://sites.google.com/metodix.fi/artikkelit/futuribles).
The catalyst in Delphi rounds is the manager or management team. The manager is responsible for the panel composition and makes choices regarding the recycling of surveys, affecting the course of the research process. Bold ways to motivate and guide the panel’s operation are acceptable, provided they are based on research objectives and the manager does not manipulate results or arguments. Research ethics are not method-dependent, but they differ when the goal is to explore multiple futures instead of one truth. Language model AI applications may even disrupt the manager’s toolkit in the near future, which will also present additional challenges in terms of research ethics.
Web technology has enabled that even in single-round Delphis (Real-Time Delphi), the phenomenon and knowledge process can iterate. The usual and traditional number of rounds is two or three. A fourth round is often a so-called feedback round, in which panelists are once again involved to evaluate the conclusions or future scenarios drawn from information accumulated by managers in earlier rounds.
The number of rounds depends on the research objectives and resources. A common process is three-phased, where the second round expands and deepens the examination of the phenomenon based on the first round, and the third round confirms the key results, analyses, and conclusions (known as the feedback round).
Fundamentally, the iterative process represents a Popperian model of evolving knowledge formation, which is the core idea of scientific activity. Even as an imperfect thought and action model, it enables the continuous accumulation of knowledge and understanding, provided the process can remain open to (new) observations and various kinds of comments, including critical ones. The task of iteration is to refine the initial data collection into feedback-driven interaction and thereby reorganize the information originally shared by panelists in a manner that can be called communal learning. As a result of learning, good decisions and wise actions are also expected to emerge. The experimental phase is underway in terms of how extensively and in which areas generative artificial intelligence in its various forms can be utilized or pose a threat.
References
- Ahvenharju, Sanna (2022) Futures Consciousness as a Human Anticipatory Capacity – Definition and Measurement. Turun yliopisto. PDF Full Text https://urn.fi/URN:ISBN:978-951-29-8892-1 .
- Airaksinen Tiina, Halinen Irmeli, Linturi Hannu (2016) Futuribles of Learning 2030 – Delphi supports the reform of the core curricula in Finland. European Journal of Futures Research. Special topic: Education 2030 and beyond. Internet https://link.springer.com/article/10.1007/s40309-016-0096-y.
- Bell, Wendell (1997, 2003) Foundations of futures studies, Vol I-II, New Brunswick: Transaction Books.
- The Delphi Technique: Past, present and future prospects. Technological Forecasting and Social Change, Volume 78, Issue 9, Pages 1487-1720 (November 2011)
- Dimitrow, Maarit (2016) Development and Validation of a Drug-Related Problem Risk Assessment Tool for Use by Practical Nurses Working with Community-Dwelling Aged. Helsingin yliopisto, farmasian tiedekunta. Helsinki. Verkossa https://helda.helsinki.fi/handle/10138/167914
- Gordon, T.J. (2008) The Real-Time Delphi Method. The Millennium Project. Futures Research Methodology – V3.0. Verkossa http://www.millennium-project.org/FRMv3_0/05-Real-Time_Delphi.pdf .
- Kauppi, Antti ja Linturi, Hannu (2018) Kansalaisfoorumin viisi tulevaisuutta. Internetissä https://metodix.fi/2018/11/30/kansalaisfoorumin-viisi-tulevaisuutta/ .
- Korhonen-Yrjänheikki, Kati (2011) Future of the Finnish Engineering Education – A Collaborative Stakeholder Approach. ISBN 978-852-5633-48-1. TEK. Miktor. Helsinki. Verkossa http://www.tek.fi/ci/pdf/julkaisut/KKY_dissertation_web.pdf.
- Koskimäki Teemu (2022) Expert perspectives on achieving global sustainability with targeted transformational change. A thesis submitted for the degree of Doctor of Philosophy of The Australian National University. PDF-julkaisu https://openresearch-repository.anu.edu.au/bitstream/1885/274587/1/TK%20-%20PhD%20thesis%20-%20Revised%20version%202022%20FINAL.pdf .
- Kuusi, Osmo (2000) Delfoi-metodi. Internetissä https://metodix.fi/2014/05/19/kuusi-delfoi-metodi/ .
- Kuusi, Osmo (1999) Expertise in the Future Use of Generic Technologies. Epistemic and Methodological Considerations Concerning Delphi Studies. Interneissä http://bit.ly/3ayzN4e .
- Laakso, K., Rubin, A. & Linturi, H. 2010. Delphi Method Analysis: The Role of Regulation in the Mobile Operator Business in Finland. Phuket, Thailand: PICMET 2010: Technology Management for Global Economic Growth. 18.- 22.7.2010, 2698-2704.
- Laukkanen, Minttu (2020) Sustainable business models for advancing system-level sustainability. Acta Universitatis Lappeenrantaensis -tutkimussarja 889. ISBN 978-952-335-470-8 ja ISSN 1456-4491. LUTPub-tietokanta www.urn.fi/URN:ISBN:978-952-335-471-5 .
- Linturi, Hannu & Rubin, Anita (2011) Toinen koulu, toinen maailma. Oppimisen tulevaisuus 2030. Turun yliopiston Tulevaisuuden tutkimuskeskus. Tutu-julkaisu 1/2011.
- Linturi, Hannu, Rubin, Anita, Airaksinen, Tiina (2012) Lukion tulevaisuus 2030 – Toinen koulu, toinen maailma. Otavan Opiston Osuuskunta. 978-952-6605-00-5 (pdf), ISSN-L 2242-1297, ISSN 2242-1297.
- Linturi, Hannu, Linturi, Jenni ja Rubin Anita (2013) eDelphi – metodievoluutiota verkossa. Metodix https://metodix.fi/2014/11/26/edelfoi-metodievoluutiota-verkossa/ .
- Linturi, Hannu, Rubin Anita (2014) Metodi, metafora ja tulevaisuuskartta. Futura 2/2014.
- Linturi, Hannu (2007) Delfoin metamorfooseja. Futura 1/2007.
- Linturi, Hannu (2017) OPH:n Oppimisen tulevaisuus 2030-barometri. Internetissä https://metodix.fi/2017/01/11/oppimisen-tulevaisuus-2030-blogi-2017/ (https://metodix.fi/2016/12/31/oppimisen-tulevaisuus-2030/) .
- Linturi, Hannu (2020) Delfoin monet tarkoitukset. Metodix https://metodix.fi/2020/03/08/delfoin-tarkoitukset/
- Linturi, Hannu (2020) Delfoi-prosessin vaiheet. Metodix
- Linturi, Hannu (2020) Delfoi-pedagogia. Internetissä https://metodix.fi/2019/11/15/delfoi-pedagogia/.
- Linturi, Hannu (2020) Ilmastot@komo: viisi työkalua ilmastokasvatukseen. Internetissä https://metodix.fi/2019/12/01/ilmastotakomo/ .Rand (2020) Delphi Method https://www.rand.org/topics/delphi-method.html
- Linturi Hannu (2023) Delfoin seitsemän ideaa. Blogisarja https://metodix.fi/2023/10/09/delfoin-seitseman-ideaa/. Metodix Oy.
- Linturi Hannu & Kauppi Antti (2021) Miten tutkimme tulevaisuuksia Delfoi-menetelmällä, Artikkeli teoksessa Delfoilla tulevaisuuteen, toim. Merja Kylmäkoski & Päivi Raino. Humak-ammattikorkeakoulu https://www.humak.fi/julkaisut/delfoilla-tulevaisuuteen/ .
- Linturi Hannu & Kuusi Osmo (2022) Tulevaisuuksia ennakoiva Delfoi-menetelmä. Artikkeli teoksessa Tulevaisuudentutkmus tutuksi. Perusteita ja menetelmiä. (toim. Hanna-Kaisa Aalto, Katariina Heikkilä, Pasi Keski-Pukkila, Maija Mäki, Markus Pöllänen). Turun yliopisto https://www.utupub.fi/handle/10024/153465 .
- Myllylä, Yrjö (2007) Logistic and Social Future of the Murmansk Region until 2020. Joensuun yliopisto, Yhteiskunta- ja aluetieteiden laitos. Joensuu. Verkossa http://joypub.joensuu.fi/publications/dissertations/myllyla_murmanskin/myllyla.pdf.
- Mäkelä, Marileena (2020) The past, present and future of environmental reporting in the Finnish forest industry. Turun yliopiston julkaisua – Annaels Universitatis Turkuensis, Ser. E: Oeconomica. URN:ISBN:978-951-29-8087-1. Verkossa https://www.utupub.fi/handle/10024/149753?show=full .
- Osaamisen ennakointifoorumi: The Finnish National Agency for Education’s Skills Forecasting Forum conducted a multi-phase development project from 2016 to 2019 to explore the future of the workforce and vocational education, employing the Delphi technique as a method to open up futures. Initially, all actors within nine sectoral clusters assessed future developments up to 2035 according to the dynamic multi-level model described by Geels and Schot. From this data, four scenarios were constructed, two of which were selected as the basis for further work. In the second Delphi round, separate Delphi processes were implemented for the nine clusters, which also were based on three levels examining changes in the operating environment, regime adaptation, and signal-level innovation phenomena. See the eDelphi main panel at https://www.edelphi.org/oef and Jukka Vepsäläinen’s video presentation at https://youtu.be/8o2v5nNWqIo?si=T1FyVIibVfX5LdSK.
- Paaso, Aila (2010) Osaava ammatillinen opettaja 2020. Tutkimus ammatillisen opettajan tulevaisuuden työnkuvasta. Rovaniemi: Lapin yliopisto 2010, Acta Universitatis Lapponiensis 174. ISBN 978-952-484-348-5. ISSN 0788-7604.
- Palo, Teea (2014) Business model captured?: variation in the use of business models. University of Oulu, Oulu Business School, Department of Marketing. PDF Full Text http://urn.fi/urn:isbn:9789526203430 .
- Pernaa, Hanna-Kaisa (2020) ”Hyvinvoinnin toivottu tulevaisuus – tarkastelussa kompleksisuus, antisipaatio ja osallisuus”. URN:ISBN:978-952-476-910-5. Osuva http://urn.fi/URN:ISBN:978-952-476-910-5
- Pihlainen, Vuokko (2020) Asiantuntijoiden käsityksiä johtamisosaamisen nykytilasta ja tulevaisuuden suunnista suomalaisissa sairaaloissa 2030. Experts’ perceptions of the present state of management and leadership competence and future directions in Finnish hospitals by 2030 Kuopio: Itä-Suomen yliopisto, 2020 Publications of the University of Eastern Finland Dissertations in Social Sciences and Business Studies; 223. ISBN: 978-952-61-3377-5 (print), ISBN: 978-952-61-3378-2 (PDF), ISSN: 1798-5757 (PDF). Verkossa https://erepo.uef.fi/bitstream/handle/123456789/22263/urn_isbn_978-952-61-3378-2.pdf
- Rand Corporation: Delphi Method https://www.rand.org/topics/delphi-method.html
- Rubin, Anita (2007) Pehmeä systeemimetodologia. Internetissä https://metodix.fi/2014/05/19/rubin-pehmea-systeemimetodologia/ .
- Rönkä, Anu-Liisa (2019) Kohti vuorovaikutteista riskiviestintää : Tapausesimerkkinä langattoman viestintätekniikan säteily. University of Helsinki, Faculty of Social Sciences. Doctoral Programme in Interdisciplinary Environmental Sciences. Internet https://helda.helsinki.fi/handle/10138/301671 .
- Soini-Salomaa, Kristiina (2013) Käsi- ja taideteollisuusalan ammatillisia tulevaisuudenkuvia. HY. Käyttäytymistieteellinen tiedekunta. Helsinki. Verkossa https://helda.helsinki.fi/handle/10138/41734
- Sunell, Otto (2016) Turvallisuuskulttuuri julkisen hallinnon organisaatiossa vuoteen 2025 tultaessa: Nykytilan kartoitus ja neljä skenaariota. Tampereen teknillinen yliopisto. Tampere. Verkossa https://tutcris.tut.fi/portal/en/publications/turvallisuuskulttuuri-julkisen-hallinnon-organisaatiossa-vuoteen-2025-tultaessa(d16cf10d-2d88-4bcb-8dad-62349f74bf36).html .
- Tamminen, Nina (2021) Mental Health Promotion Competencies in the Health Sector. Faculty of Sport and Health Sciences, University of Jyväskylä. Permanent link to this publication: http://urn.f/URN:ISBN:978-951-39-8666-7 .
- Tapio, Petri (2002) The limits to traffic volume growth : The content and procedure of administrative futures studies on Finnish transport CO2 policy. Internetissä https://helda.helsinki.fi/handle/10138/22446 .
- Tapio, Petri (2002) Disaggregative policy Delphi Using cluster analysis as a tool for systematic scenario formation. Technological Forecasting and Social Change.
- Toivonen, Annette (2022) The emergence of New Space – A grounded theory study of enhancing sustainability in space tourism from the view of Finland. Acta electronica Universitatis Lapponiensis 336. ISBN: 978-952-337-311-2, ISSN 1796-6310. University of Lapland, Rovaniemi 2022. Sähköisen julkaisun pysyvä osoite: https://urn.fi/URN:ISBN:978-952-337-311-2 .
- Turoff, Murray (2002) The Delphi Method, Techniques and Applications. Internetissä https://web.njit.edu/~turoff/pubs/delphibook/delphibook.pdf .
- Valtonen, Vesa (2010) Turvallisuustoimijoiden yhteistyö. Maanpuolustuskorkeakoulu, taktiikan laitos. Julkaisusarja 1, Helsinki. Verkossa http://www.doria.fi/handle/10024/74154 .
Kategoriat:artikkeli, Artikkelit, blogi, Tie
Jätä kommentti